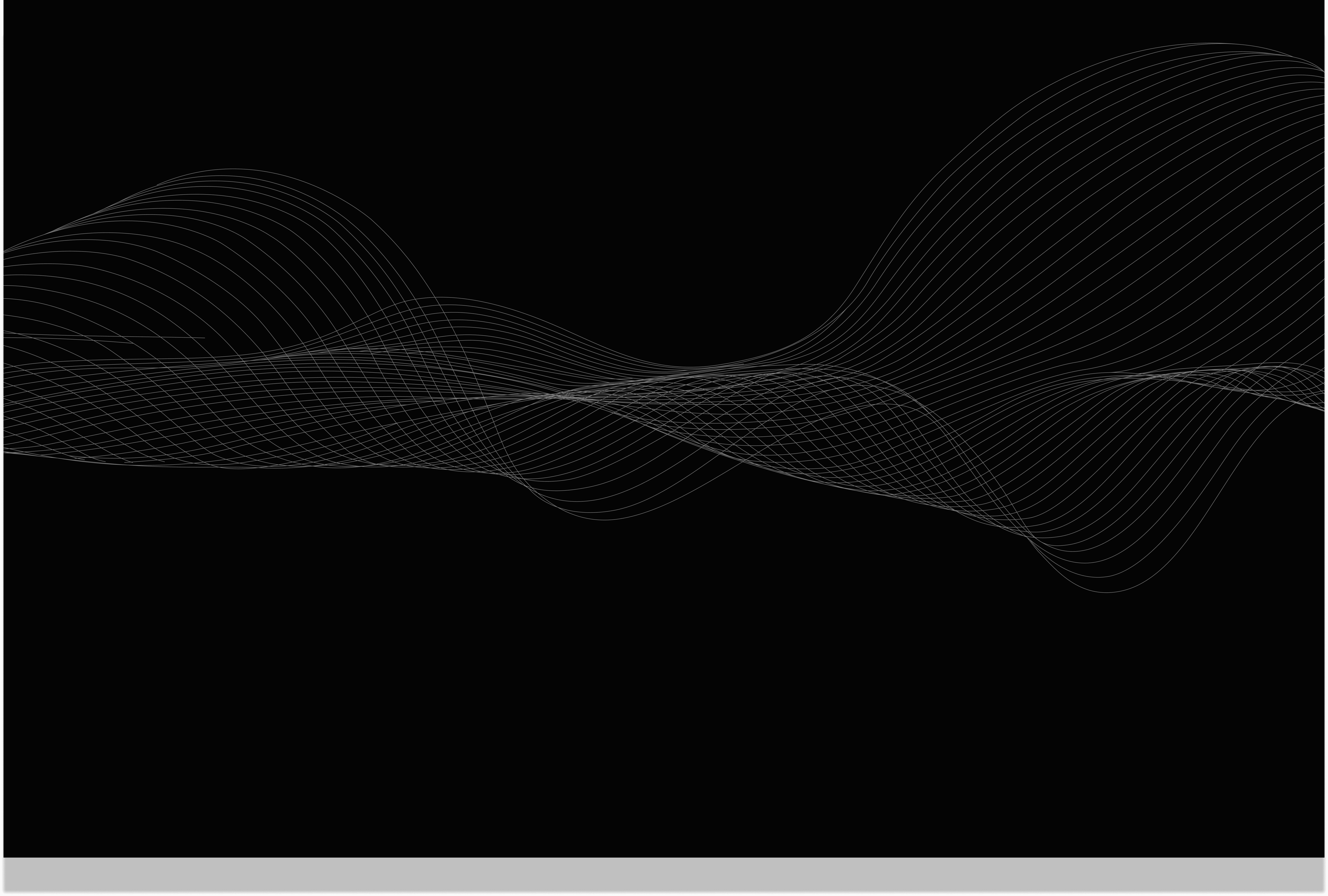
How to Optimize Your Voice AI Stack for the Financial Industry
Apr 29, 2025
The Unique Challenges of Voice AI in Finance
1. Numerical Precision and Pronunciation
Financial conversations often revolve around precise numerical information:
Account balances that must be communicated accurately to the cent
Transaction amounts that cannot be misrepresented
Market prices and percentages that require exact pronunciation
Date formats that vary across regions but must be consistently interpreted
A single misinterpreted digit in a financial context can lead to significant errors and customer frustration. Voice agents must be trained to pronounce numbers, decimal points, and currency symbols with absolute clarity and in formats familiar to users.
2. Strict Instruction Following and Compliance
Financial services operate under rigorous regulatory frameworks:
Voice agents must follow precise protocols for customer verification
Compliance disclosures must be delivered verbatim in many cases
Agents need to understand when to escalate to human representatives
Scripted responses may be required for certain financial products
Unlike conversational AI in other domains, financial voice agents often need to adhere to specific language and process requirements that leave little room for creative interpretation.
3. Security and Authentication Challenges
Voice interactions in finance require robust security measures:
Voice biometric authentication integration
Secure handling of sensitive personal and financial information
Protocols for confirming identity without compromising privacy
Clear communication about security processes to build trust
4. Domain-Specific Financial Terminology
Finance has its own complex lexicon:
Industry jargon and specialized terms (APR, ETF, LIBOR, etc.)
Product names that may be difficult to pronounce
Regulatory terminology that must be accurately represented
Financial abbreviations and acronyms that require proper vocalization
5. Emotional Intelligence During Financial Stress
Financial conversations often occur during moments of customer stress:
Discussions about financial hardship require appropriate tone and empathy
Fraud alerts and account issues need to be handled with both urgency and calm
Financial decision-making assistance must balance facts with sensitivity
Optimizing Your Voice AI Stack: Essential Components
1. Speech-to-Text (STT) Optimization for Finance
When evaluating STT solutions for financial applications, consider:
Numerical accuracy: Test the system's ability to correctly transcribe various numerical formats, including:
Currency amounts with decimals
Account numbers
Percentage figures
Dates in multiple formats
Financial domain training: Consider providers that offer domain-specific models or fine-tuning capabilities to recognize financial terminology.
Accent and dialect coverage: Ensure the STT component performs well across your customer demographics, particularly with numbers spoken in various accents.
Real-time correction mechanisms: Implement confirmation loops for critical numerical information to verify accuracy.
2. Language Model Selection and Optimization
The LLM component should be evaluated for:
Instruction adherence: Test the model's ability to follow strict protocols and scripts when required for compliance.
Financial knowledge: Assess the model's understanding of financial concepts, products, and regulations.
Contextual awareness: Evaluate how well the model maintains context about account information and customer history throughout a conversation.
Guardrails implementation: Ensure robust safeguards against providing financial advice beyond the agent's authorized scope.
Customization potential: Consider models that allow fine-tuning or specialized prompt engineering for financial use cases.
3. Text-to-Speech (TTS) Requirements for Financial Communication
Key considerations include:
Numerical pronunciation clarity: Test how clearly the voice pronounces numbers, decimal points, and financial symbols.
Pacing control: Ensure the system can adjust speaking rate for important information like terms and conditions or account numbers.
Emotional appropriateness: Verify the voice can convey the right tone for different financial contexts - professional for technical information, empathetic for financial hardship discussions.
Brand alignment: Consider custom voices that reflect your institution's brand identity and values.
4. Turn Detection and Conversation Management
Financial conversations often require:
Interruption handling: Customers may need to interject during lengthy explanations or to correct information.
Extended response accommodation: Some financial responses may be longer than typical conversational turns.
Silence tolerance: Customers may need time to check information or consider options during financial discussions.
Questions to Ask As You Build & Scale Your Voice AI Application
1. Use Case Prioritization
Where can we reduce friction in existing customer journeys?
Which transactions are high-volume but straightforward enough for voice AI?
What are the escalation criteria for transitioning to human agents?
2. Compliance and Security Assessment
How will we ensure our voice AI adheres to regulatory requirements in all jurisdictions we serve?
How can we make sure our voice AI adheres to the required authentication methods for users?
3. Integration Strategy
What latency requirements are acceptable for different financial transactions?
What fallback mechanisms will we implement when voice interactions fail?
4. Testing and Evaluation Framework
How will we measure accuracy for financial information communication?
What compliance metrics should we track for regulatory reporting?
How can we test the system across diverse customer demographics?
What benchmarks will indicate successful deployment across different financial tasks?
Scaling Your Financial Voice AI: Advanced Considerations
1. Multi-Modal Integration
As you scale, consider how voice AI interfaces with:
Mobile banking applications for visual confirmation
SMS for secondary verification of transactions
Email for follow-up documentation
Customer relationship management systems for context awareness
2. Progressive Complexity Implementation
Start with simpler financial tasks before advancing to more complex ones:
Begin with account balance inquiries and transaction history
Progress to internal transfers between accounts
Advance to bill payments and external transfers
Eventually handle more complex advisory or product recommendation functions
3. Continuous Learning Infrastructure
Implement systems for:
Analyzing common failure points in financial conversations
Identifying new financial terminology or product names that require updating
Tracking regulatory changes that require voice agent retraining
Monitoring customer satisfaction specific to financial interactions
4. Redundancy and Reliability Planning
Financial services demand exceptional reliability:
Implement backup providers for critical stack components
Design graceful degradation paths for system limitations
Establish clear business continuity processes for voice system outages
Monitor end-to-end latency for time-sensitive financial operation
Evaluating Your Financial Voice AI: Comprehensive Testing Framework
Testing voice AI in financial contexts presents unique challenges that require specialized evaluation methodologies. Financial voice AI demands a more rigorous testing approach than other domains due to the high stakes of potential errors.
Component-Level Performance Evaluation for Financial Use Cases
Each component of your financial voice AI stack requires specialized evaluation metrics:
Speech-to-Text (STT) Financial Metrics
Financial Word Error Rate (F-WER): Specialized WER that weights errors on financial terms and numbers more heavily
Numerical Transcription Accuracy: Specifically measuring precision on currency amounts, account numbers, and percentages
Financial Terminology Recognition: Accuracy on industry-specific terms, product names, and regulatory language
Multi-accent Performance on Financial Terms: Testing across customer demographics with financial vocabulary
LLM Financial Performance Indicators
Compliance Adherence Rate: How accurately the model follows regulatory scripts and disclosures
Financial Information Accuracy: Correctness of product information, rate calculations, and policy details
Decision Logic Consistency: Reliability of the model's financial recommendations and responses
Sensitive Information Handling: Appropriate management of personal financial data
TTS Financial Quality Metrics
Numerical Pronunciation Clarity: How accurately and clearly the voice pronounces financial figures
Financial Term Pronunciation: Correctness on industry terminology and product names
Appropriate Tone Modulation: Ability to adapt voice characteristics to sensitive financial discussions
Information Pacing: Appropriate speed adjustments when delivering critical financial information
End-to-End Financial Conversation Metrics
Transaction Completion Rate: Success percentage for common financial tasks
Error Recovery Effectiveness: How well the system recovers from misunderstandings in financial contexts
Escalation Appropriateness: Correctness of decisions to transfer to human agents for complex cases
Financial Voice AI Testing Challenges
Voice AI evaluation for financial applications faces several unique difficulties:
High-stakes outcomes: Financial errors can have significant consequences for customers
Compliance verification: Ensuring adherence to complex regulatory requirements
Financial edge cases: Rare but critical scenarios that must be handled correctly
Demographic inclusivity: Ensuring equitable performance across all customer groups
Building Financial Voice AI Evaluation Systems with Coval
To overcome these challenges, leading financial institutions are turning to specialized evaluation platforms like Coval that provide:
Financial synthetic datasets: Pre-built and customizable conversation flows that represent specific financial use cases, from simple balance inquiries to complex mortgage discussions
Domain-specific metrics: Comprehensive evaluation frameworks designed specifically for financial voice AI that measure what truly matters in banking and investment conversations
Continuous performance monitoring: Real-time tracking of key financial conversation metrics with alerts for performance degradation
Component benchmarking: Comparative analysis of different providers for each stack component based on financial performance criteria
Automated regression testing: Regular validation of voice AI performance against a library of financial conversation scenarios
Coval's platform allows financial institutions to:
Compare STT providers specifically on financial terminology recognition
Benchmark LLMs on compliance adherence and numerical reasoning
Evaluate TTS solutions for clarity on critical financial information
Track end-to-end performance on complete financial customer journeys by simulating end users and catching issues before they go live
Financial Voice AI Metrics: Solving the Hard Problems
The most challenging aspects of evaluating financial voice AI are precisely where specialized platforms provide the greatest value:
Financial conversation datasets: Access to diverse samples across various financial scenarios, customer demographics, and conversation complexities
Financial success metrics: Clearly defined measures that correlate with both compliance requirements and customer satisfaction
Risk-appropriate testing: Frameworks that balance thoroughness with practical implementation timelines
Financial edge case libraries: Comprehensive collections of unusual but critical financial scenarios
Financial institutions that invest in robust evaluation infrastructure with specialized platforms like Coval establish a foundation for trusted, high-quality voice experiences that meet both customer expectations and regulatory requirements.
Conclusion
Building an effective voice AI stack for the financial industry requires balancing technical performance with the unique demands of financial services - precision, compliance, security, and emotional intelligence. By addressing the specific challenges of financial conversations and implementing a thoughtfully designed evaluation framework, financial institutions can leverage voice AI to enhance customer experiences while maintaining the trust and reliability that define successful financial relationships.
As financial institutions scale their voice AI implementations, the complexity of evaluation increases exponentially. Platforms like Coval provide the specialized testing infrastructure needed to ensure voice agents perform reliably across all financial contexts. By investing in comprehensive evaluation strategies tailored specifically to financial use cases, institutions can accelerate development while maintaining the rigorous standards that financial services demand.
As voice technology continues to advance, financial institutions that invest in properly optimized and rigorously tested voice AI stacks will gain significant competitive advantages through improved customer satisfaction, operational efficiency, and service accessibility. In an industry where trust is paramount, the quality of your voice AI evaluation framework may ultimately determine your success in this rapidly evolving space.